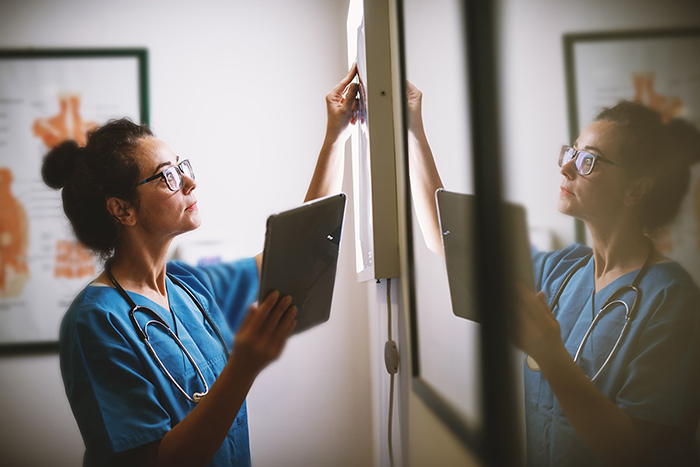
Many of us have seen sci-fi movies in which a “medical scanner” diagnoses and treats everything from rapid aging to chemical exposure. Today’s healthcare providers would welcome such a device or system to offer fast diagnoses, treat or cure diseases, and even prevent health problems. Scientists and engineers are already pioneering new tools and methods to advance healthcare in revolutionary ways. Support from agencies like NICHD enable these enterprising researchers to test promising ideas that could improve patient care. The following advances are just a few examples of emerging technologies funded by NICHD.
Reducing time-to-diagnosis in hospitals
Diagnosis can be the most important step in healthcare. While some conditions are fairly straightforward to diagnose, other conditions may be misdiagnosed or undiagnosed for days or even years. For vulnerable patients like newborn babies, diagnostic delays or failures can be life-threatening.
In a study published in April 2019, researchers described a better method to diagnose rare diseases in infants admitted to intensive care units. The research team developed a faster whole-genome sequencing method and paired it with an artificial intelligence system that could quickly sift through genomic data and healthcare records. The method reduced the average time-to-diagnosis from 16 days to about 20 hours. Having a diagnosis by the next day, as opposed to two weeks, can make a life-saving difference for patients and their families.
Diagnosing jaundice, a common health condition among newborns, typically relies on healthcare providers detecting abnormal yellowness in the skin or whites of the eyes. But this form of diagnosis isn’t always accurate and can be especially problematic in low-resource areas around the world. In 2017, NICHD-supported researchers published work on a smartphone app that analyzes digital images to diagnose newborn jaundice. The app relies on a form of artificial intelligence called machine learning, in which computer software automatically learns from its own experience. The app does not require extra equipment and can be used in the home or clinic, making this tool especially useful in low-resource areas.
Sepsis is another serious condition for which prompt diagnosis and treatment can save lives. NICHD-funded researchers are applying machine-learning techniques to diagnose sepsis more quickly and accurately. In April 2018, researchers reported a new, experimental test that could diagnose sepsis by measuring the mobility of neutrophils, a type of immune cell, in a single drop of blood. NICHD also recently awarded two grants that will use machine learning to diagnose sepsis in infants and children. Both projects (HD096402 and HD096961) aim to use machine learning to distinguish between different subtypes of sepsis. Refining diagnoses in this manner will help healthcare providers tailor treatments so that patients receive optimal care, quickly.
Improving healthcare in the home and clinic
Technological advances aren’t limited to hospitals; they also are available for use at home or in non-emergency settings like doctor’s offices and clinics.
Many people are familiar with urine-based home pregnancy tests (for which NICHD developed the technology back in the 1970s) and home ovulation testing kits, which allow women to obtain results in the privacy and comfort of their own homes. In December 2018, NICHD-supported researchers described a better way to test for ovulation at home. They created an inexpensive device that uses a smartphone and artificial intelligence-based software that looks for patterns in a drop of saliva. The new test is reusable, making it cheaper over time compared to one-time use sticks for urine tests. The saliva-based test also is better for women with certain gynecological conditions, such as polycystic ovary syndrome, that involve hormonal imbalances and make urine-based tests less accurate.
Normally, diagnosing autism spectrum disorder (ASD) requires screening tests done by trained professionals that take hours to administer and evaluate. Diagnosis delays are common for children who live in rural areas, are from a racial or ethnic minority, or are from a lower socioeconomic status. Two recent studies applied machine learning to streamline ASD screening in children. In one study, published November 2018, researchers used mobile devices with special software to analyze short, 2-minute home videos. Using this method, an ASD diagnosis took about 4 minutes. In the other study, published in April 2019, researchers described a new automated tool to diagnose ASD in toddlers. The new method is faster, eliminates human bias, and does not require clinical training to perform, according to the study team.
Modeling health conditions and diseases
Accurately modeling a disease or condition can also help healthcare providers improve diagnoses and better predict treatment outcomes. Artificial intelligence and other technologies are particularly well-suited to this type of predictive modeling.
For example, NICHD-funded researchers developed a model back in 2010 that helps predict the chances of a live birth after a couple receiving fertility treatments experience a failed in vitro fertilization (IVF) attempt. Such information could help couples and physicians decide whether or not to try another IVF cycle.
Modeling also is useful for treating stroke, for which timely and careful decisions by healthcare providers are critical for a patient’s recovery. Large-scale modeling of stroke would enable researchers to understand how to best treat a patient depending on the type of brain damage or lesion caused by the stroke. However, such studies are hard to conduct because analyzing lesions from brain imaging is not an automated process. Even with training, it can take several hours to trace one complex stroke lesion. NICHD-supported researchers created a tool to automate this process. ATLAS (Anatomical Tracings of Lesions After Stroke) is a large, free database of traced stroke lesions that research teams can use to test the accuracy of their experimental software and models.