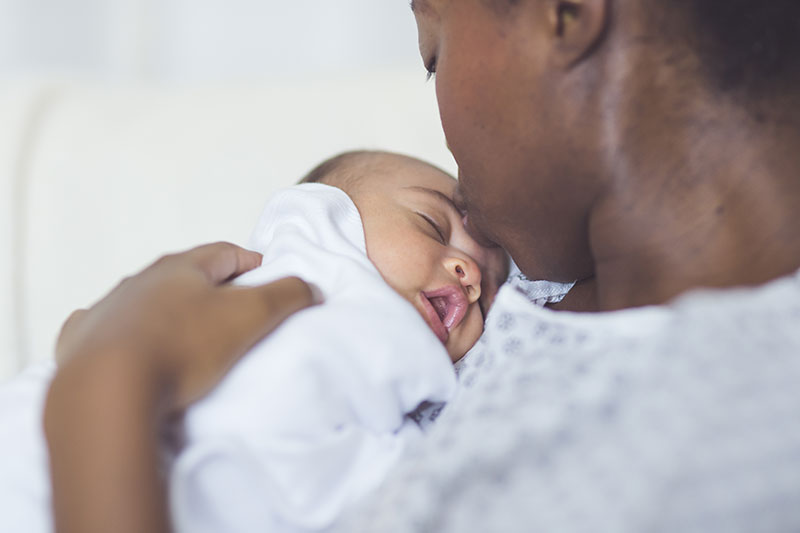
Computational analysis of the word usage in personal stories of recent birthing experiences can identify women likely to develop posttraumatic stress disorder (PTSD) related to childbirth, suggests a study funded by the National Institutes of Health. The findings may aid development of a low-cost screening tool to help diagnose and treat childbirth-related PTSD.
The work was funded by NIH’s Eunice Kennedy Shriver National Institute of Child Health and Human Development (NICHD) and led by Sharon Dekel, Ph.D., of Massachusetts General Hospital. It appears in the American Journal of Obstetrics & Gynecology MFM.
Background
Worldwide, an estimated 6% of people who give birth will experience postpartum PTSD related to their delivery experience. This translates to approximately 8 million cases of childbirth-related PTSD in 2022. In addition to undermining maternal welfare, untreated postpartum PTSD impedes mother-infant bonding and can lead to emotional and physical neglect of the child.
Early identification of childbirth-related PTSD would help ensure that new parents receive the treatment and support they need. Although screening for postpartum depression is routine in the United States, there is no recommended protocol to identify people likely to experience childbirth-related PTSD. Additionally, many new parents hestitate to report postpartum mental health symptoms to their healthcare providers due to shame, stigma, or fears of being separated from their infants.
The researchers theorized that the text in a narrative recounting an individual’s recent birthing experience may provide insights into the person’s risk for developing childbirth-related PTSD. They developed a model based on natural language processing (NLP) and machine learning to analyze the word usage in such narratives. NLP-based technologies enable computers to understand text in much the same way human beings can, while machine learning enables computer systems to automatically learn from experience without being explicitly programmed.
Results
The researchers used their NLP model to evaluate short, unstructured accounts of the recent childbirth experiences of 995 postpartum women enrolled in a research study about childbirth during the COVID-19 era. Participants also completed a PTSD symptom screening survey.
The narrative-analysis method accurately identified 80% of women who met the provisional diagnostic criteria for childbirth-related PTSD and 70% of women who did not develop the condition. The scientists also found that participants who developed childbirth-related PTSD used more words to describe their birthing experiences, compared to those who did not develop PTSD. The narratives of women who developed PTSD also included fewer positive emotions, more negative emotions, and more body- and death-related words.
Significance
The study suggests that computational analysis of personal childbirth narratives generated in the early postpartum period can distinguish women who are likely to develop childbirth-related PTSD from those at low risk. Such short written narratives could be collected remotely ahead of a medical visit, so that appropriate care could be provided to those identified as being at higher risk for childbirth-related PTSD.
Next Steps
Additional studies are needed to validate the results and evaluate ways to further improve the accuracy of prediction, such as by combining personal narratives with information in patients’ medical records. Because most participants in the current study were middle-class American women, the authors note that future work should include more diverse populations. They also suggest that the method could be applied to explore racial and ethnic disparities related to childbirth-associated trauma.
Reference
Bartal A et al. Identifying women with post-delivery posttraumatic stress disorder using natural language processing of personal childbirth narrative. AJOG MFM DOI: 10.1016/j.ajogmf.2022.100834 (2022)